Hey hey everyone, how are you this fine ThursdAI? 👋 I’m gud thanks for asking!
I’m continuing my experiment of spilling the beans, and telling you about everything we talked about in advance, both on the pod and in the newsletter, so let me know if this is the right way to go or not, for the busy ones it seems that it is. If you don’t have an hour 15, here’s a short video recap of everything we chatted about:
ThursdAI - Jan 11 2024 TL;DR
TL;DR of all topics covered + Show notes
Open Source LLMs
🔥 Donut from Jon Durbin is now top of the LLM leaderboard (X, HF, Wolframs deep dive and scoring)
OpenChat January Update - Best open source 7B LLM (X, Hugging Face)
Our friends at NousResearch announce a seed round of 5.2M as their models pass 1.2 million downloads (X)
Argilla improved (Distillabeled?) the DPO enhanced Neural Hermes with higher quality DPO pairs (X)
New MoEs are coming out like hotcakes - PhixTral and DeepSeek MoE (X, Omar Thread, Phixtral Thread)
Microsoft makes Phi MIT licensed 👏
Big CO LLMs + APIs
OpenAI adds personalization & team tiers (Teams announcement)
OpenAI launches GPT store (Store announcement, Store link)
Mixtral medium tops the LMsys human evaluation arena, is the best LLM overall after GPT4 👏 (X)
Hardware
Rabbit R1 is announced, $200/mo without a subscription, everybody has a take (X)
This weeks Buzz from Weights & Biases
Video
Bytedance releases MagicVideo-V2 video gen that looks great and passes Pika labs in human tests (X)
AI Art & Diffusion & 3D
Luma launched their online version of Genie and it's coming to the API (X)
Show notes and links mentioned
MergeKit (github)
Jon Durbins Contextual DPO dataset (HuggingFace)
Phixtral from Maxime Lebonne (X, HuggingFace)
WandGPT - out custom Weights & Biases GPT (GPT store)
Visual Weather GPT by me - https://chatg.pt/artweather
Ask OpenAI to not train on your chats - https://privacy.openai.com/policies
AI Hardware
It seems that the X conversation had a new thing this week, the AI hardware startup Rabbit, showcased their new $200 device (no subscriptions!) at CES and everyone and their mom had an opinion! We had quite a long conversation about that with
(his first time on ThursdAI 👏) as we both pre-ordered one, however there were quite a few red flags, like for example, GPUs are costly, so how would an AI device that has AI in the cloud just cost a 1 time 200 bucks??There were other interesting things they showed during the demo, and I’ll let you watch the full 30 minutes and if you want to read more, here’s a great deeper dive into this from
.UPDATE: Ss I’m writing this, the CEO of Rabbit (who’s also on the board of Teenage Engineering, the amazing company that designed this device) tweeted that they sold out the initial first AND second batch of 10K unites, netting a nice $2M in hardware sales in 48 hours!
Open Source LLMs
Mixtral paper dropped (ArXiv, Morgans take)
Mistral finally published the paper on Mixtral of experts, the MoE that's the absolutel best open source model right now, and it's quite the paper. Nisten did a full paper reading with explanations on X space, which I co-hosted and we had almost 3K people tune in to listen. Here's the link to the live reading X space by Nisten.
And here's some notes courtecy Morgan McGuire (who's my boss at WandB btw 🙌)
Strong retrieval across the entire context window
Mixtral achieves a 100% retrieval accuracy regardless of the context length or the position of passkey in the sequence.
Experts don't seem to activate based on topic
Surprisingly, we do not observe obvious patterns in the assignment of experts based on the topic. For instance, at all layers, the distribution of expert assignment is very similar for ArXiv papers (written in Latex), for biology (PubMed Abstracts), and for Philosophy (PhilPapers) documents.
However...
The selection of experts appears to be more aligned with the syntax rather than the domain
Datasets - No info was provided to which datasets Mixtral used to pretrain their incredible models 😭
Upsampled multilingual data
Compared to Mistral 7B, we significantly upsample the proportion of multilingual data during pretraining. The extra capacity allows Mixtral to perform well on multilingual benchmarks while maintaining a high accuracy in English
Mixtral Instruct Training
We train Mixtral – Instruct using supervised fine-tuning (SFT) on an instruction dataset followed by Direct Preference Optimization (DPO) on a paired feedback dataset and was trained on @CoreWeave
Jon Durbin Donut is the 🤴 of open source this week
6 of the top 10 are donut based models or merges of it. If you remember Auroborous, Donut includes that dataset, and there are two varieties there, the DPO and the non DPO versions of Bagel, including two merges from Cloudyu, which are non trained merges with mergekit, based on Donut.
Jon pro tip for selecting DPO vs Non DPO models is
FYI, the DPO version is more factual, truthful, better at math, etc., but is not great for RP, creative writing, etc. Use non-DPO for those tasks!
Donut includes an impressive amount of dataset mixed together, which are all linked from the model card but here they are:
"ai2_arc, airoboros, apps, belebele, bluemoon, boolq, capybara, cinematika, drop, emobank, gutenberg, lmsys_chat_1m, mathinstruct, mmlu, natural_instructions, openbookqa, pippa, piqa, python_alpaca, rosetta_code, slimorca, spider, squad_v2, synthia, winogrande, airoboros 3.1 vs airoboros 2.2.1, helpsteer, orca_dpo_pairs"
Jon also shared his end of the year WandB report nad has trained a whopping 917 models this year for a total of ~2500 hours and is in the top 10% of the top active users (among 800K or so users)
I didn't know that Jon is going to join, but was so happy that he joined the live recording that we ended up chatting for 20 minutes, and there was so many nuggets in that conversation, about how to prepare DPO datasets, which other ones Jon has been releasing, and just a bunch more gold, that I decided to CUT that out and post it as a separate special deepdive episode that's going to get released on the Sunday special. Stay tuned for that!
Nous Research announces $5.2 million funding seed round as they cross 1.1 million model downloads on the hub
Congrats to Karan, Emozilla, Teknium, Bowen, Shivani and the rest of the Nous team on this great news!
👏 We expect to hear more from them in the coming year, with a consistent commitment to open source, keep open sourcing the best models, and the upcoming Forge news!
With investors like Balaji, OSS capital, Vipul from Together, Nous completes the $5.2M seed round, and we had Karan (one of the co-founders of Nous) on the pod to chat to use about what they are planning to do with that money and what are their continuous commitments to open source!
In addition, they just recently passed 1.1 million downloads on the hub with Nous-Hermes-2-34B being their best model! 🤴
OpenChat Jan update becomes the leading open source 7B model (X, Hugging Face)
This update mainly enhanced training methodology, in-context learning & coding skills, outperforming the last 1210 release on 7 out of 8 benchmarks! and scores 71.3 on HumanEval, 65.8% on MMLU 👏
The previous version of OpenChat trails just behind OpenHermes on the human evals on Lmsys arena, but both are incredible 7B models.
Argilla
- Argilla used their Distilabel tool to build a preference dataset from ratings and critiques of AI response pairs, taking around 3 hours
- The original dataset assumed the GPT-4/3.5 responses were always best, but Argilla found this was not always the case
- Their dataset confirmed ~4,000 pairs had the same rating, 7,000 pairs were unchanged, and ~2,000 times the rejected response was preferred
- Improving existing DPO datasets with higher quality pairs is important for model fine-tuning
- They are releasing an improved version of the popular Orca Pairs DPO dataset from Intel, and a new OpenHermes model outperforming baselines with 54% fewer DPO pairs
Big CO LLMs + APIs
OpenAI has a big week, launches GPTs store and team pro accounts (Blog)
Things of note about the store:
My GPTs are getting feedback and crossed 10K chats , was #6 on lifestyle and the disappeared, but has gained 2x more chats in 24 hours since the store has launched!
Discoverability is great, trending GPTs are shown clearly, and folks are getting a lot of exposure
Copycats already started copying a bunch of the great GPTs, see this example of what happens when you search for Gymstreak, most of the top GPTs are already being copy-catted.
Team accounts:
$25/mo per user for annual plans and at least 2 teams
The biggest confusion was from folks who didn't understand that OpenAI trains on Pro conversations, and there's an option to Opt-out!
This weeks Buzz (What I learned with WandB this week)
Weights and Biases (and ME!) are going to AGI house to lead a Rag vs Finetune hackathon with cool prizes!
There's still time to RSVP, will incredible guests speakers, this Hackathon is organized together with... LangChain, TogetherCompute and AGI house - If you're in the SF area, and you wanna hack on some cool RAG things and get awesome prizes (and meet me!) join the waitlist here https://partiful.com/e/AlntdLtxh9Jh1J6Pcsma
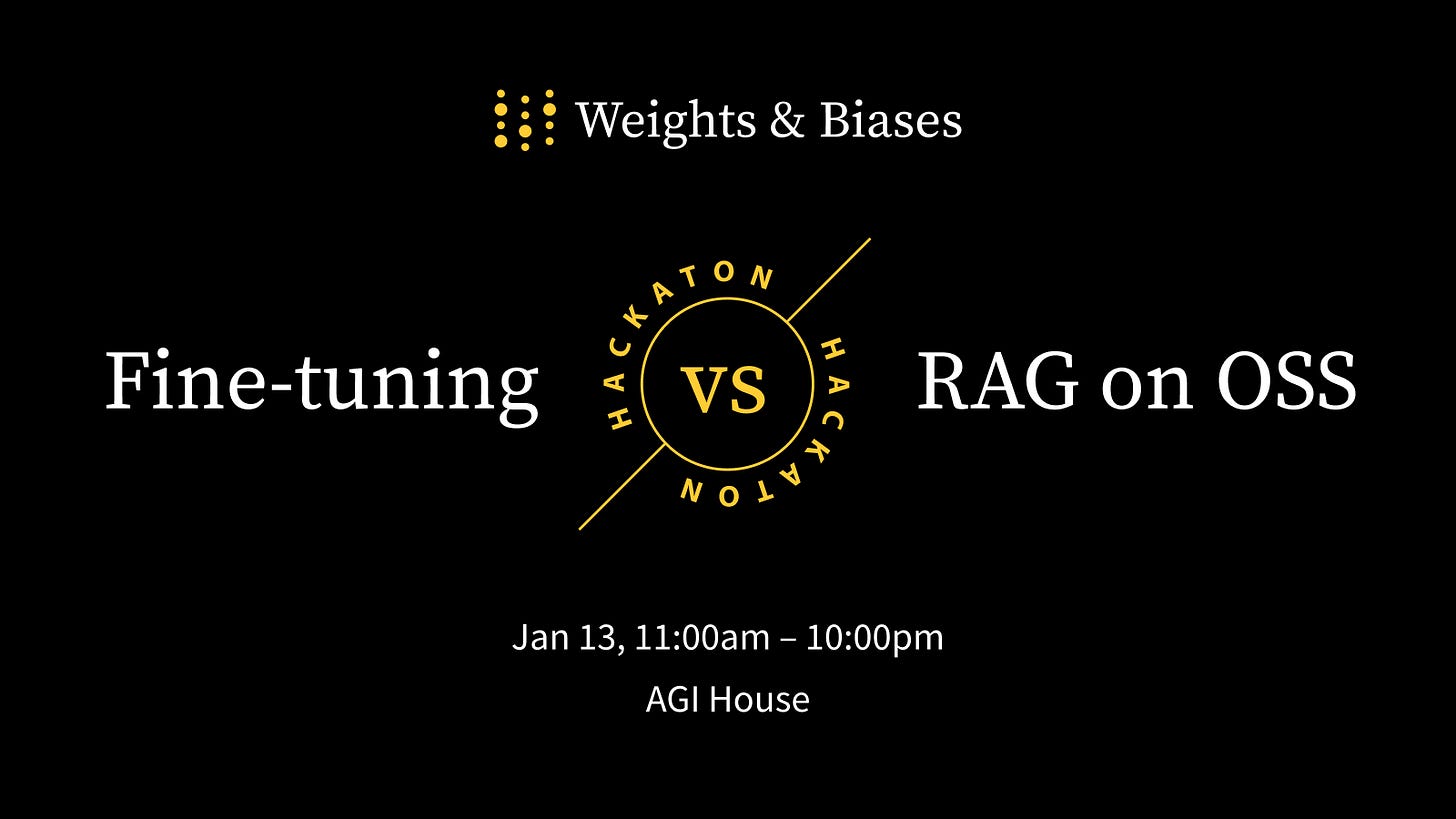
Vision & Video
Luma released GENIE on Web and IOS, if you remember, we covered the GENIE text-to-3d model they first released on discord a while ago, and now it's incorporated into the luma website, and is significantly higher quality 3D assets.
The generations are free for now, and they look awesome! Here are some of mine, I created a Bee holding a Wand (get it? WandB? 😆) and a polish bear (internal joke) and they look so cool!
Friend of the pod and recent LUMA hire Arthur Islamov jumped on and also told us that this is coming to the API, so developers would be able to automate asset creation and generate tons of 3D objects programmatically, and use cool prompt techniques to make sure they are a bit better every time maybe? Great news!
AI Art & Diffusion
Bytedance announces MagicVideo-V2 (Arxiv, Project)
We didn't get anything besides quite a few cherry picked videos and a paper, so we can't use this yet, but wow some of these videos look incredible!
MagicVideo-V2 that integrates the text-to-image model, video motion generator, reference image embedding module and frame interpolation module into an end-to-end video generation pipeline. Benefiting from these architecture designs, MagicVideo-V2 can generate an aesthetically pleasing, high-resolution video with remarkable fidelity and smoothness. It demonstrates superior performance over leading Text-to-Video systems such as Runway, Pika 1.0, Morph, Moon Valley and Stable Video Diffusion model via user evaluation at large scale
Lastly, I had the greatest time to interview my new friend João Moura, the creator of Crew AI, which been popping off, was the #1 trending on Github and #2 of the day on Product hunt, and is essentially an AI framework that lets you create a crew of AI agents to do tasks for you. I will be polishing up that conversation and post it together with the deep dive with Jon, so stay tuned, but here’s a sneak preview of how cool this is and expect that episode to drop soon!
For convenience here’s a full transcript with timestamps:
ThursdAI - Jan 11th
[00:00:00] Alex Volkov: Let's get started with ThurdsAI. Hopefully
[00:00:08] Alex Volkov: you guys are familiar with the sound by this time. Welcome everyone. Welcome to ThurdsAI. Our weekly AI episode covering the latest and greatest in updates from the open source AI scene, LLM's vision, audio, sound. This week we have some hardware to chat about that kind of broke the Twitter sphere and everything else that we deem very cool and important in the world of AI for the last week. , I will just say for everybody who joined us for the first time my name is Alex Volkov. I'm an AI evangelist with Weights Biases. And I've been very happy to Host these spaces weekly and this is a live recording of our podcast.
[00:00:54] Alex Volkov: The podcast is also called ThurdsAI, it's also a newsletter and you are more than welcome to subscribe to it if you haven't yet. Just in case you miss any of the two hours show that we have for you today you'd be able to quickly recap. , if you need to run, if you have a Thursday meeting, Thursday is usually busy for many people we send a newsletter. It's thursdayi. news, this is the URL, and you get all the show notes as well there, as well as like different multimedia stuff, like videos and different things.
[00:01:22] Alex Volkov: More than welcome to subscribe to that.
[00:01:24] Alex Volkov: Here's a little bit of editing magic for you. This is Alex from the editing room, telling you that the next section is a 7 minute excerpt from the end of the live recording, recapping everything we talked about, so you could hear things in the past tense. This is the best recap of the two hours that are going to follow and you're welcome to listen to this and if any part interests you, you're welcome to skip ahead and listen to that part.
[00:01:56] Alex Volkov: So I hope you enjoy and let me know in feedback if this works for you.
Mitral releasing the Mixtral of Experts paper
[00:02:00] Alex Volkov: So, we started with talking about Mixtral and Mistral released a paper about mixture of experts and we've talked with Omar and about what mixture of experts actually is.
Bagel with Jon Durbin
[00:02:10] Alex Volkov: We've talked about Bagel , which is now taking the top leaderboard in OpenLLM in HuggingFace. And we actually got the pleasure of Jon Durbin, the author of Ouroboros and then Bagel datasets and models to join us and talk about how he creates them, what's DPO, what's the difference. It was super cool.
[00:02:26] Alex Volkov: A great conversation. If you missed that definitely worth a release and it's going to get released on ThursdAI pod, very soon.
OpenChat January update
[00:02:33] Alex Volkov: We also mentioned the open chat release, the January update, which makes it on the human eval and MMLU scores the best 7B LLM out there.
Nous Reserach $5.2M seed round and OSS commitment
[00:02:41] Alex Volkov: We've talked about our friends from Nous Research announcing a seed round of $5.2 million which is super cool. We had Karan, the co-founder . of Nous Research talked to us about the plans to use the seed money and the fact that, the plan to, the commitment to open source remains and they plan to keep releasing stuff, unlike some other companies that raise and then stop releasing stuff in open source.
[00:03:01] Alex Volkov: So we really appreciate Nous Research. I personally, am wearing a Nous Research T shirt right now, and I'm very happy for this friendship, and Nous always has a place on ThurdsAI.
Argilla Distil Labeled NeuralHermes and improved it with less DPO pairs
[00:03:12] Alex Volkov: We also mentioned Argilla or Argilla and they have a Distilled Label feature, they, I think, open sourced on GitHub as well, and they improved the DPO set that was used to train the neural Hermes.
[00:03:24] Alex Volkov: Just a brief refresher. There's the open Hermes from Teknium from Nous Research, then folks from Intel ran a synthetic data set on top of this and they, they call it neural Hermes, which improved the score. And then now there's the fine tune of a fine tune of a fine tune. Argilla improved that DPO with some of their techniques and then released like a even the higher score with less.
[00:03:46] Alex Volkov: DPO pairs so like just with less data sets, it was super cool.
Omar covered MoE architecture, Phixtral and DeepSeek MoE
[00:03:49] Alex Volkov: Omar Sanseviero the Chief Lama Officer from HuggingFace, keeps joining us, I love it. He's an expert in many things, specifically he talked about the MOE architecture, he talked about two cool things, he talked about Phixtral so Phixtral is the way to pronounce this, the Phi model from Microsoft was just recently MIT licensed, probably this week as well, and already Maxime Le Bon created the mixture of experts on top of Phi, which is super cool.
[00:04:14] Alex Volkov: And he also mentioned we also talked about merge kit and the ability to merge models with this merge kit and Omar covered the deep seek MOE that was released less than 12 hours ago with the paper. And also this is a full. pre trained model, this is not a fine tune of a different model. This is not Frankenstein merge.
[00:04:33] Alex Volkov: DeepSeek released like a pre trained MOE and worth checking out. And DeepSeek Coder, we mentioned last week, was one of the best coder models around.
Big Companies segment
[00:04:41] Alex Volkov: Then, we moved to the big companies area.
Mistral Medium is now the best LLM after GPT4c
[00:04:44] Alex Volkov: And this week was the first time that I put Mistral both in the open source segment and in the big company segment, because Mistral is offering a non open source API for their Mixtral Medium, which just is exciting what the large will mean, but their Mixtral Medium, apologies, we don't know the size of this, we only have estimations, but we saw this week that LMSYS Arena with over 6, 000 votes, folks prefer Mixtral on top of other LLMs.
[00:05:16] Alex Volkov: And this is essentially the best LLM after GPT 4 overshadowing, Cohere Anthropic like all these companies that we've talked about before this company Mistral from Europe that started this summer folks who started Mistral, I think from DeepMind and the original Lama. Paper, some of them are, I think Guillaume Lempel is on the original Lama paper.
[00:05:36] Alex Volkov: They released a bunch of cool stuff and now they're literally like the second best in the world and in all of them, not only proprietary, et cetera. So just trailing behind the three versions of GPT 4. So Mistro is now becoming a big company with APIs as well. I suggest you use
OpenAI big week, personalization, Team tiers and GPT STORE
[00:05:52] Alex Volkov: this. Then we talked about OpenAI.
[00:05:54] Alex Volkov: Releasing personalization, which allows the GPT to remember the stuff that you told it from a new chat to a new chat and a new chat, and it's super cool. And I think personalization is definitely a trend that we're going to see in 24, hopefully we'll move to open source as well.
[00:06:07] Alex Volkov: And we also talked about their team tier announcement where you and some of your friends could spend a little bit more money, but get twice as much quota. And then also not have OpenAI trained on your inputs. It's really cool for teams as well. Hopefully our team in Weights Biases will add me to the team tier as well.Uh And also they have
[00:06:27] Alex Volkov: released the GPT store finally. GPT store is their marketplace for custom GPTs that showcases and highlights the trending ones and the most used ones and the ones that I released, Visual Weather, just crossed 5, 000 chats, which is super cool. It was trending for a while on the GPT store. We also talked about the issue with Copycats and many copycats on GPD store starting to pop up. So something that new that OpenAI is going to have to deal with and we'll see their commitment to deal with this like marketplace on top of everything else OpenAI does now they have to run the marketplace and also like every marketplace, every app store comes with its own sort of problems.
Alex in SF for the Together, Langchain and WandB hackathon in AGI house
[00:07:03] Alex Volkov: We then, I mentioned that I'm going to be in San Francisco. Flying tomorrow, hopefully if my flight, the cancelled one, is going to get replaced to do a hackathon with LengChain and TogetherCompute and WeightsAndBiases in AGI house. So if you're in the area, more than welcome to register. The link will be in the show notes.
[00:07:20] Alex Volkov: And I would love to see you there. We're going to Try and hack together a solution, or at least a discussion about what's better, RAGing systems or fine tuning models, and then running your application on top of them, or maybe both, or maybe neither. We're going to talk about, and we're going to hack together around all this with some cool prizes.
[00:07:36] Alex Volkov: I'm going to try to do What I always do when I do on trips, I give you the little taste of San Francisco when you're not in San Francisco. So follow me for live streams from there.
Bytedance MagicVideo
[00:07:46] Alex Volkov: We mentioned the ByteDance releases magic video.
Luma labs releases ERNIE on web, text to 3D
[00:07:49] Alex Volkov: And then we also mentioned LumaLabs released their online version of Genie which is a text to 3d.
[00:07:54] Alex Volkov: It's super cool. You just type text and you get a 3d model, which is like incredible. We live in like unprecedented cool times.
Interview with Joaão Moura from CrewAI
[00:08:01] Alex Volkov: We then had a great pleasure of talking with João Moura. The author of Crew ai, which is a. Agent framework in the open source based on lang chain currently Python is rewritten in TypeScript that is able to run your agents on top of closed models.
[00:08:16] Alex Volkov: So whatever GPT 4 and even probably [00:08:20] Mistral or Medium, the one that I just mentioned, but also has support for Ollama and support for LM studio and is able to run those agents using completely offline, completely on your device LLMs, such as he specifically mentioned the Nous Hermes model and other models as well.
[00:08:38] Alex Volkov: Some of the fine tuned models that we've talked about here. And João is a super cool dude. Definitely give him a follow and listen to the whole conversation because it was great to hear why in the field of Already existing AI agent frameworks, he decided to build another one and then he became like the number one training project on GitHub and number two product on Product Hunt
Rabbit R1 AI harware device announced on CES
[00:08:58] Alex Volkov: And then to Finish this all off. We had Dan Jeffries, and we've talked about the Rabbit R1, the hardware device, which has a built in OS, custom device that doesn't do apps, but only has AI built into there, designed by Teenage Engineering, recently launched on CES and had many folks get excited and not excited.
[00:09:19] Alex Volkov: People were saying, Hey, I don't want this. People saying, yes, we do. We want this. And we shared our opinions about it. What seems fake or what could be exciting and the fact that, obviously in ThursdAI, we come here with optimism and we want things like this to succeed. And so I definitely pre ordered one and that conversation was also great.
[00:09:36] Alex Volkov: Thank you, Dan. Thank you, Umesh, for that.
[00:09:37] Alex Volkov: And if any of what I just mentioned interests you, I think you'll enjoy the full conversation, the full two hour conversation with the authors of the things we've mentioned with the experts as always on ThursdAI this is when I take my drink.
[00:10:06] Sounds: ThursdAI music
Mixtral of experts paper
[00:10:08] Alex Volkov: All right. I think the most Interesting, not the most interesting thing, but definitely worth chatting about is the Mixtral paper. So a Mixtral paper called Mixtral of Experts Mixtral, the fine folks in Mixtral published this paper and also hosted, which unfortunately I wasn't able to attend. They hosted a, an hour in their discord and I know some folks here attended that.
[00:10:30] Alex Volkov: It was super cool. And Mixtral of Experts, as we've talked about last week is The best open source model bar none in the world right now, the best MIT licensed model. It's the best performing both on like on the LMC Serena and human evaluation is definitely up there. It's, it passes Anthropic Squad.
[00:10:51] Alex Volkov: And I think it's just behind Anthropic One, which for some reason Anthropic is, getting worse. The more models they release, the less they're getting voted on. And so this is. The best model that's MIT licensed. Everything else after it for a while is also non commercially licensed.
[00:11:08] Alex Volkov: And they go a little bit into depth for how they train the model. Unfortunately, they don't give us any Information about the pre training and data sets. So they don't talk about this. They don't talk about what the experts are necessarily, but they cover several things.
[00:11:27] Alex Volkov: And here's some of my things. I would love to hear from the folks here on stage who probably listened to this paper as well. I will just mention that Nistan, who's a frequent co host in ThurdsAI, and I had a paper reading when I say, and I, Nistan did 95 percent and I was just there to like, Help him like, take a breath.
[00:11:44] Alex Volkov: But basically did a full paper reading on Live on X and I will add this to the show note. It was super, super cool. And people who joined us like really enjoyed it. So maybe we're going to do more of those. Mr. Lito read the whole paper. I'm going to explain a bunch of the concepts and I learned a bunch from this.
[00:11:58] Alex Volkov: So I'll definitely add this to the show notes for you guys. Here's the summary also from Morgan from Weights and Biases, who's my boss that I took some stuff. Strong retrieval across the entire context window. Mixtro was released with 32, 000 tokens in the context window and it had strong retrieval With almost a hundred percent, I think they mentioned a hundred percent retrieval accuracy, regardless of the context length or the position of the passkey.
[00:12:25] Alex Volkov: So they did the needle and haystack analysis that we've talked about multiple times before. And in the paper, they mentioned that they have a hundred percent accuracy in retrieval, which is really incredible. If you guys remember John Carbride? I don't know how to pronounce his name. Did the needle and haystack analysis for GPT.
[00:12:45] Alex Volkov: For Turbo when it came out with 128k tokens, and then for Cloud as well, and then did kind of pressure testing and you probably if you've been following Thursday for a while, you can probably visualize this like Excel of green stuff and then a bunch of like red dots. This is usually the problem with like longer context windows.
[00:13:07] Alex Volkov: That accuracy is not actually there. And Tropic came out and said, Hey, you're prompting this wrong. If you add this like specific thing, the accuracy goes from 27% to 97% something crazy. And so it's great to see that, first of all mixed roll is referring to the accuracy on their longer contact window, but also do they have a hundred percent retrieval, which is super cool.
[00:13:28] Alex Volkov: The other thing that they mentioned in the paper, which wasn't that. Long, the paper itself, is that experts don't seem to activate based on topics specifically. They went fairly deep into kind of how experts activate, and they have, they talk about how they have a, access to two experts per token and this creates a significantly better model.
[00:13:49] Alex Volkov: They compared to Lama a bunch, to Lama70B. And they said that we beat Llama70B at pretty much everything, including being a smaller model, to be able to run on a smaller model, including the as you inference, the model is actually like significantly smaller as well. But they did mention, they did go into the whole mixture of experts thing, and they said that they didn't observe patterns of assignment to experts based on topics.
[00:14:11] Alex Volkov: I think they took eight sections of the pile data set, the huge data set, Wikipedia section, and then philosophical paper section and then archive and PubMed for biology and some of it was written in latex and then, oh, Phil papers, the philosophy papers. And then they didn't notice that any specific expert is getting activated per specific like section of Of the pile data set, which is interesting because when you hear the name mixture of experts and hopefully Omar will dive a little deeper into this in his segment, when you hear a mixture of experts, you imagine, a math whiz and a coding expert, et cetera, all different like experts and the model just like routes to a specific expert, but it doesn't sound like this is what they have on the on the actuality.
[00:14:54] Alex Volkov: The, There was something aligned with syntax more than the knowledge as well, which was very interesting. So they did notice that syntax, for example, keeps sending tokens to, to more of the same expert more than the domain of the knowledge. Could be because of the huge data sets that go into this.
[00:15:12] Alex Volkov: Data sets were not talked about. And so we didn't get information about the pre training or data sets at all. One thing that's worth mentioning significantly is that they mentioned on the paper, and I think that this signi like, this strongly shows how Mistral is becoming the open source alternative, at least in Europe, is that they've pre trained on a bunch of multilingual data as well, and they have show strong performance in all of the European top languages.
[00:15:38] Alex Volkov: So German, Italian, Spanish and I think French, obviously French. Obviously, the company is from France. And they significantly add this information in the pre training. And it's definitely becoming The open source if in the European market, that's why I think that in my head and in my predictions for 24, Mistro is going to be the open source of Europe.
[00:15:59] Alex Volkov: And I think that's most of the notes that I'm taking from Morgan's tweet. Umesh or Alignment, did you guys read the paper? What'd you guys think? Is there anything important that I didn't cover yet?
[00:16:11] Umesh Rajiani: For me the biggest thing was the conclusion where they have you already mentioned about the tokens. It was very interesting to see the how tokens change at different layers. So they have shown the zero layer, 15th layer, and 31st layer. And that was the most interesting part because then if we are looking at the mixture of experts architecture as such where MOE Mamba is also in the make, in the making, then the whole premise activating experts basically goes out, out of the [00:16:40] window.
[00:16:40] Umesh Rajiani: If that, the activation mechanism is different. So that was the most interesting part for me. Yeah.
[00:16:46] Alex Volkov: Yeah, absolutely. Thanks so much. And Omar, hopefully you rejoined us what's your takeaway, a brief takeaway from the Mixtral paper? Something that you may be didn't see before.
[00:16:56] Omer Sanseviero: Eh, so to be honest, I don't think it shared anything that the community didn't know already. Quite a bit of this literature around what expertise means. So for example, that the different experts. Become express more implementation or birth or different parts of the sentence. That's something that was already showed in previous papers.
[00:17:18] Omer Sanseviero: So I don't think the paper provided anything that we didn't know already. I think dataset would be, would have been quite nice.
[00:17:26] Alex Volkov: Awesome. Thank you. Thank you, Omar.
LMSys released an arena Update placing Mixtral Medium just behind GPT-4 variants
[00:17:28] Alex Volkov: Yeah. All right. So this is Mixtral. Moving on, I think it's worth mentioning here before we move on that, I think I mentioned this briefly. LMSys released an update and they collect 6, 000 upvotes and the Mixtral medium, which we don't know, it's only rumored how big this is, right? Mixtral, the one that we got is eight, eight experts of 7 billion parameters.
[00:17:50] Alex Volkov: All are Mixtral 7b or at least initiated from Mixtral 7b. The Mixtral medium is only available in API, right? So we've covered previously that Mistral also released an API solution. You can register to the console, get an API key and start basically OpenAI did for a while and Anthropic does, and then they have another offering there.
[00:18:09] Alex Volkov: That they call medium. So I wonder what large is. So we don't know the size of it. There's rumors that it's 14B, 8X plus of 14B. There's rumors that it's like 8X plus of 30B. I'm not sure if we know, I don't think they said. However, based on LMsys and evaluations, it looks like this is the best model Sorry, the second best model in the world of LLMs right now, just behind the GPT 4.
[00:18:34] Alex Volkov: It's literally like number four in the LMsys arena, but like the first three is GPT 4 and all its versions, right? The March version, the June version, and then the recent I think it was November version. So all the three top ones are GPT 4 Turbo from November, and then and then the June version, and then the March version, and then the fourth one is Mixtral Medium.
[00:18:56] Alex Volkov: I'm sure they're working on a large one, and I don't know if that's gonna pass GPT 4, but it's really interesting that until we get to see Gemini, that supposedly beats GPT 4, at least in some things Mixtral, or from Mistral, the guys who just opened the company in the summer, is now the second best LLM in the world.
[00:19:15] Alex Volkov: I think it's worth shouting out as well, and kudos for LMsys for giving us this evaluation. And, I haven't yet played with the API myself as well. I really want to get to a point where I have a UI that can plug that API in. So hopefully I'll find one soon. I think we're covering Mistral and we're moving forward.
Bagel models the leaderboard from Jon Durbin
[00:19:32] Alex Volkov: We're moving forward into the top open source on the LLM leaderboard and the creator. So if you guys open the open source LLM leaderboard, which we often talk about. On HuggingFace we've talked about the difference between human evaluation and the automatic evaluations that OpenLLM leaderboard runs.
[00:19:52] Alex Volkov: You will see a bunch of models. The top three ones are from CloudU and they're like, I think merges of Yee34 and then the Mixtroll34b as well, but it's not based on Mixtroll. And then the rest of the is like a bunch of John Durbin Bagel examples. And, so all of those, there's six models there that are based basically on the John's Bagel DPO versions.
[00:20:20] Alex Volkov: And I just wanted to shout this out and shout out John Durbin for working this hard and releasing these models.
[00:20:26] Alex Volkov: Let's see if we can hear from the man himself. Hey, John.
[00:20:29] Jon Durbin: Hey, how's it going?
[00:20:29] Jon Durbin: Yeah, I'm a software engineer. I'm an AI tinker. I've been doing synthetic stuff since I guess maybe April with Aragoros project. It's been tons of fun. Lately I've been mostly working on the bagel models. If you're wondering what the bagel name came from, it's from Everything, Everywhere, All at Once.
[00:20:45] Jon Durbin: Great movie.
[00:20:47] Alex Volkov: Hey, Alex from the editing floor here again, interrupting your flow here. What follows is a 20 minute conversation of one of the best fine tuners and dataset creators around, current king of the leaderboards, John Durbin of Ouroboros and Bagel fame. And that full conversation, including another deep dive, will be released as a follow up episode.
[00:21:10] Alex Volkov: I decided to just Give it justice and put it on its own episode. So if that interests you, make sure that you follow Thursday Eye and you'll get it in your email in a few days as a special episode. So continuing with our next topic.
Conversation with John on a special episode of THursdAINous Research announces seed round $5.2M and commitment to open source
[00:21:23] Alex Volkov: I wanna mention that. Very good friends of the pod, and I consider like multiple folks here, personal friends of mine already at this point.
[00:21:31] Alex Volkov: Nous Research announced some news this week, and I have Karan here, who's the founder and technium in the audience, and unfortunately not the whole team. Shivani couldn't make it because of some other stuff. We've talked with Nous Research before they were cool. That's what I love to say.
[00:21:45] Alex Volkov: And obviously you guys know Tekken, he's new Hermes data set and Hermes are probably the best kind of fine tunes of 7B and many people use them, they're part of Together Compute as well. Nous Research announced a seed round of Quran, please correct me if I'm wrong, 5. 2 million. From a bunch of investors and Balaji Srinivas I think, and OSS Capital and, We pull from together and a bunch of other folks and quite an impressive kind of resume of investors.
[00:22:13] Alex Volkov: I'm going to add this to the show notes for you guys as well. I just wanted to welcome Karan and give you the chance to say a few words and congratulate you myself. And as I'm wearing and sitting here, I'm wearing the new Swissers blue t shirt that I have since that event in San Francisco. That we've met and had a lot of fun.
[00:22:29] Alex Volkov: Welcome Karan, congratulations. What's the plan for all the seed money?
[00:22:35] Karan: Thank you so much for for having me, first of all, Alex, and thanks for bringing some light to Noose Research, as always, and the open source work that we love to do. I'd love to give a little context in that. Noose really started as a volunteer project. We still have a massive amount of volunteers, and it started from a group of people who have worked in open source and watched companies close off their technology after getting funding.
[00:23:01] Karan: We've seen that happen time and time again, where a company turns its back and says, we're going to go fully private now. A huge motivation for us was saying that, we have to have some bastions and places that are always going to maintain. And Open Source Philosophy and Open Source Releases to allow people to iteratively develop so we can continue to catalyze the speed of work.
[00:23:24] Karan: First and foremost, it has a lot to do with bringing some of our most passionate and dedicated members to full time work. Because everybody who's worked on the MUSE project has done it in their free time. And we wanted to take a small clade of people and be able to let them do it full time. So with one piece of what we're going to do with the seed money, it's we're going to pump more and more resources into open source work.
[00:23:49] Karan: We're going to keep dropping Hermes models. We're going to keep dropping multimodal models. We're going to be doing everything that we can. Simultaneously, I'm sure you have seen some demos. In SF and on Twitter of the Noose Forge that's currently in a very early state. We are developing a sort of software development kit for agent building and local inference that can all be utilized on local compute.
[00:24:14] Karan: So that's something that we are very excited to bring everybody. We want to be able to build basically an area where you can build all your tools. Where you can do all of your inference, where you can have all your interactions with various different agents, where you can build all of your automation pipelines.
[00:24:32] Karan: That's very important for us to create one hub where all of the different pieces from data synthesis to inference to utilizing agents, pretty much everything besides training can go down. So that's a little bit behind what we're actually trying to build and give out to devs. But all of the open source work will continue and only ramp up from here as well.
[00:24:54] Alex Volkov: That's. So great to hear. This was my next question, so thank you for covering this in advance, Karan, and I have [00:25:00] only this to say.
[00:25:02] Alex Volkov: Congrats, congrats on the raise, on the effort brother, congrats everybody in news. I wanna like huge shoutout for first of all, not closing off and continuing the open source stuff.
[00:25:11] Alex Volkov: It's very important for the community and I love that statement is written in advance specifically with the background of OpenAI not being like super open. And also I wanna congratulate you on passing 1 million downloads. I think you guys are 1. 1 on HuggingFace under the Nous Research Umbrella with 44 models and Nous Hermes 2 34B is being like the best model on, on, on the leaderboard right now.
[00:25:33] Alex Volkov: And expecting like incredible, great things from you. As always, ThurdsAI is a great stage for you guys to always join and talk about the stuff that you release. Can't wait to play with Forge personally and and keep representing Nous Research. Huge congrats. Anything else you want to say?
[00:25:47] Alex Volkov: Maybe where folks can come and help feel free to invite the community to participate more.
[00:25:52] Karan: Yeah, absolutely. We started and continue as a massively volunteer project and anything we do from people we just anonymously meet can transform into a full paper as it has before. So please feel free to stop by on our Discord, which is available in the link in the bio for Atnoose Research. So if you just go to Atnoose Research on Twitter, you can join our Discord and we'd love to collaborate with all of you.
[00:26:18] Karan: Thanks again, Alex, and thank you to the whole ThursdAI family
[00:26:21] Alex Volkov: thanks for joining, man. Thank you. Thank you. And everybody who works on very hard on releasing like the best fine tuning models.
Open Chat January update - 71 Human Eval, 65% MMLU - best 7B benching finetune
[00:26:26] Alex Volkov: All right, we're moving forward. I'll briefly mention some of the stuff because we don't have that much time and Omar, I think you had the most interesting segment next.
[00:26:34] Alex Volkov: I just want to mention briefly the OpenChat release their January update. OpenChat definitely. Every time the release, like the model becomes a little better. They're not renaming the model as well. Open chat this January is just the January update. It has it beats the previous update in I think December 10th of seven out of the eight benchmarks it beats like a previous update.
[00:26:55] Alex Volkov: I don't think it's, I'm not sure if it's DPO or not. It scores 71 percent on human eval. And 65 MMLU, definitely like significant scores for a 7b model. And really performs well on the LMC Serena as well. I think they're like trailing just behind the OpenNermus and this is with the previous model.
[00:27:12] Alex Volkov: So the new model that was just released worth mentioning will be linked in the show notes. Alayman, you want to mention anything about this, the latest release of OpenChat? I know friends are
[00:27:21] Alignment Labs: just that it's not for anyone who's curious it's not DPO'd, it's actually trained using CRLFT, which is, I'll post the paper below, but this is the paper that the engineer who works on that wrote about a conditional training technique that allows you to train on mixed quality data, and the model will actually outperform if you just trained on the best data you had.
[00:27:41] Alex Volkov: Awesome. I'm going to, I'm going to add the. The model to show notes, as always, this model is already on Hugging Face Hub. I think it's part of Hugging Chat. And then, yeah, it's on Hugging Chat already. I think it's quantized already. You can, you guys definitely can use this in Elm Studio. It looks like and in other places.
[00:27:57] Alex Volkov: So shout out to OpenChat. And I think Omar, you want to chat with us about some of the exciting MOE stuff that's been happening around the open source world?
MoE introduction from Omar Sanseviero
[00:28:07] Omer Sanseviero: Yes, for sure. Yeah. So I think there have been two exciting releases this week. So one is fixed trial and the other one is deepy, MOE. Before chatting about them I will take just two or three minutes to talk a bit more about Moe in general. So just taking a step back and chat a bit about them. So the first thing is you mentioned this in the menstrual paper, is that there's a bit of confusion that Moes are a bit like ensemble of models. Which is not exactly the same. So when we talk about expertise, it's not really that each expert is becoming an expert in a different topic. So yeah, let me just quickly talk about MOIs.
[00:28:44] Omer Sanseviero: So there are two different goals when people started to do MOIs. So the first one is to do very efficient retraining. So let's say that you have 10, 000 or 100, 000 hours of GPU time. If you use an MOE you will achieve a better model if even so it can be through more data or through larger size with a fixed amount of compute, or you can achieve the same quality as attempts model much faster.
[00:29:11] Omer Sanseviero: So the idea of MOE is really to be very efficient when pre-training model in terms of how much compute you are spending. And the second use case or second goal is that when you have a use case with very high throughput. So if you have an API. In which you have 20 million users at the same time.
[00:29:29] Omer Sanseviero: MOEs are very nice because they map very nicely to hardware. So you can distribute and have each expert in a different GPU and that works very nicely. For local usage actually it's not so nice. I know that the community, the Hacker community has been able to do pretty much everything with 2, 3, 4 bit quantization.
[00:29:48] Omer Sanseviero: It's not really the use case of MOEs as they were designed, but the quality is quite nice. And how this works in practice is that if you look at the usual transformer model of a decoder you have multiple layers, right? And within each layer or each decoder block, you will have some attention layers, some feedforward layers, that kind of stuff.
[00:30:07] Omer Sanseviero: With the MOEs, what you do is that you replace the forward layer with a mixture layer or a sparse layer. You can And the idea is that instead of one forward layer, you will have a certain number of experts. So in the case of Mixtral, for example, it was eight. So you would have eight different forward layers in a mixture of expert layer in the case of Mixtral, but you could have more.
[00:30:33] Omer Sanseviero: And then you also have a small additional network, which is called the gate or the router, which is in charge of determining to which of these experts you would send the token. Now when we talk about passing the token to a an expert it's like a very important point. So usually a gated mechanism is in charge of sending different tokens to different experts, not different topics.
[00:30:56] Omer Sanseviero: So data state distinction or confusion. So let's say that the input is hello word. So maybe hello would go to the experts one and two, but word would go to the experts two and four and this routing. So learning. To which experts you would send the token, that's something that is learned during the pre training.
[00:31:16] Omer Sanseviero: So the goal is really not to make each expert an expert in a task, but just to handle certain types of tokens with a certain, by a certain expert, or it can be multiple experts that you activate at the same time. So Mixtral does top two expert triggering, so they trigger two experts for every token. So Mixtral as an example, in total, the model is actually quite large. So it has 47 billion parameters. But since only two two of the experts trigger, you technically just have 12 billion activated parameters if you just talk to experts. The math is a bit weird because really not all of the parameters, so not the 47 billion parameters are expert parameters.
[00:31:58] Omer Sanseviero: It also has things like attention layers and that kind of stuff, which also which is shared. So that part, this, that is not part of the, let's say, nature of expert stuff. So that's what a mixture of expert is. So now let's jump into Fixtral. So Fixtral is the same thing that so conceptual,
[00:32:14] Alex Volkov: just want to make sure that like we pause here and say thank you for this intro folks, a mixture of experts, like it's been popping off, mixture all released, like the main one, there are assumptions that GPT 4, there's like rumors that GPT 4, the significant attribution to why it's better is also a mixture of experts, I think this came from latent space bot with George Hudson, And so thanks so much for the deep dive.
[00:32:36] Alex Volkov: I definitely thought that every expert there is a specifically domain expert, but definitely this is not the case it looks like. And then the fixtural thing that comes is from the word Phi, right? P H I from Microsoft, that this week was also licensed MIT. I think very well worth mentioning that the Phi is a very The best small model that we have, it's funny to say small large language model, but this is essentially, Phi is two billion parameters, I believe.
[00:33:01] Alex Volkov: And somebody took that and started doing a mixture of experts based on, initialized based on Phi. Is that correct, Omar?
[00:33:08] Omer Sanseviero: Yeah
[00:33:09] Alex Volkov: so tell us about Fixtural and then the DeepSeek stuff.
[00:33:13] Omer Sanseviero: sure yeah. Just one thing before jumping into that something that is also a bit confusing about Mixtral is the name because it's Yeah, I think they call it [00:33:20] eight so it's a bit confusing because it's not that you have eight experts, you actually have eight experts for every MOE layer, but you have many MOE layers, so technically you have many experts.
PhixTral
[00:33:29] Omer Sanseviero: But yeah, so the first cool thing about Fixtral, I think, is the name. I think it's very catchy and I think it also helped to make it very popular so that was nice. And I don't know if you are familiar with MergeKit, I know that you mentioned it before, but it's a tool to merge. Models and pretty much all of the top models in the Hogan Faith leaderboard are using this, and merging is a weird thing because it has been studied by academia for four or five years and it has been done in computer vision since 10 years ago, maybe, and the discover communities and the thinkers communities are rediscovering some of this literature and using it in yeah, to hack very interesting things.
[00:34:05] Omer Sanseviero: And merging is very different than MOE. So merging is a, Just for example, if you have two models, which are exactly the same size, you can just average their weights or you could interpolate layers of, between the two models or you could do even weird things, such as concatenating layers, so increasing the size of the model, and that's called Franken merge if you have heard about those.
[00:34:28] Omer Sanseviero: So that's the idea of merging. And MergeFit, which is this tool, it's an open source tool that everyone is using to merge models they have an experimental branch, which is called MOE. And it's used to build MOEs. I call them MOE merging because it's a bit different. So the idea is that you pick existing models and all of them have the same architecture.
[00:34:47] Omer Sanseviero: So the same shape so they are fine tuned models and they will replace some of the forward layers with a MOE layer that will replace with multiple forward layers of these other models. And there's this very cool person called Maxime Lapon, I suggest you to follow him in Twitter. He published a very nice blog post about how to use MergeKit, by the way.
[00:35:07] Omer Sanseviero: And he merged,
[00:35:09] Alex Volkov: He did a bunch of stuff with this recent explosion. It's really cool.
[00:35:13] Omer Sanseviero: Yeah, he merged two PHY based instruct models. So one is PHY DPO, which is a DPO trained model, so it's a nice chat model. And then there's Dolphin 2. 6, which is this uncensored model. And he pretty much built a MOE using this mixed trial experimental branch in MergeKit to build this model, which was quite powerful.
[00:35:34] Omer Sanseviero: So that's pretty much it. What is funny about it is that the two base models that they used use different prompt formats. So it's quite interesting. Maybe something interesting also to mention here is that Before we said that the H expert is not an expert in a task, maybe when you do MOE merging, it's actually the case because what you're doing is that when you add these models, when you do the MOE, you need to specify which kind of prompts you want each model to handle.
[00:36:05] Omer Sanseviero: So you actually do, for example, if you have an expert that is very good with programming and you have an expert which is very good. With chat conversations, you could technically train the gating mechanism for each type of task. So it's something that you could technically do now with this very experimental stuff, if that makes sense.
[00:36:25] Alex Volkov: Interesting. So like mixture of experts is not experts in domain yet, but with merge stuff, it does go into that direction potentially.
[00:36:33] Omer Sanseviero: Yeah, exactly.
[00:36:34] Alex Volkov: Awesome. So FixedTrail from Maxim Lebon, folks. It's added to the show notes. Definitely check this out. Check Maxim out, Maxim's work and give him a follow. MergeKit is, we're going to add this as well.
[00:36:44] Alex Volkov: MergeKit is from it looks like CG123, I don't know, oh Charles Goddard, and shoutout Charles as well. The merge kit has been popping off and definitely most of the, if not all of the top leaderboard are now merges, including the stuff that we've talked to from John.
[00:37:00] Alex Volkov: So there's like the bagel stuff and then the top three models right now are merges of bagel and something else. With no continued like training, I think even Omar, I think there's also the deep six stuff, right? That we want to cover deep sick. We've talked about deep sea coder, and then we've talked about wizard LM or wizard coder that will stop like fine tune on top of deep sick.
DeepSeek MoE
[00:37:19] Alex Volkov: This is like one of the more performant coding open source that we have currently, and they just released yesterday. I didn't even have a chance to look at it, but I know you have a deep dive. Let us know what was that about?
[00:37:29] Omer Sanseviero: Yeah, so I think the model is maybe 12 hours old or something like that. So it's very recent. Yeah, so they published the models. They published the paper as well. This is a new pre trained model, so that's pretty cool, it's important to highlight that, it's very different when someone releases a fine tuned model versus a pre trained model.
[00:37:50] Omer Sanseviero: So this is forget about the merging stuff. Let's go back to the usual mixed trial kind of MOE, so the traditional MOE setup. So that's what they did to pre train the model but they did two different interesting tricks. So the first one is called fine grained expert segmentation. So pretty much let's say that you have a model so one, one of these experts they will segment it or fragment it into a specific number of fine grained models.
[00:38:18] Omer Sanseviero: So they will split the forward layer of the expert into multiple layers. And this allows each expert to be a bit more of an expert, let's say, so they will be smaller models, but they will have more specialization on what they are solving. And what is interesting is that maybe you can split them, rather than having just eight experts, you could have 100 experts.
[00:38:42] Omer Sanseviero: And you can activate a higher number of experts at the same time, but you don't increase the amount of compute that you're spending because the amount of parameters is exactly the same. So that's the first trick they did, and the second one is called shared expert isolation. So one issue that you have usually in MOEs is that you can have redundant experts.
[00:39:01] Omer Sanseviero: That's when multiple experts end up with exactly the same knowledge, so they are redundant. So what they do is that they isolate experts that are redundant, and then they set up shared experts that are always activated. So again, in Mixed Reality, you would only trigger or activate two experts. In this kind of DeepSeq MOE setup, you would activate maybe 10 or 20 experts.
[00:39:24] Omer Sanseviero: I don't know the exact number of experts I would need to look again into the code, but yeah, I think that's quite interesting. So they did a 2B model first that worked quite well. So then they scaled up to 16 billion parameters. That's the, those are the models that they open sourced today. And although the model is 16.
[00:39:42] Omer Sanseviero: 4 billion parameters, only 2. 8 billion parameters activate. So that's quite cool. And that outperforms the Lemma27B model across most benchmarks. It does not outperform Mistral7B. But it's quite interesting that you can have a large ish model just activate a very small number of parameters. And thanks to all of this specialization and redundancy removal, they are able to get some very good metrics. And this is particularly interesting if you have multiple GPUs and just like very high usage. So if you have an API service, you could do something like this.
[00:40:19] Alex Volkov: Yeah, go ahead. I think I wanted to mention that Mik Stroll mentioned this in the paper that MOE Also lends itself to scaling to some extent there's benefits when you want to run like on multiple GPUs as well, and there's downsides on running like on a single tenancy or something like this.
[00:40:39] Alex Volkov: So it looks like not only there's like benefits on running smaller number of parameters from a bigger model. There could be benefits on, and maybe this is the reason why GPT 4 was going this way and Mixtro is going this way, that there's some benefits in terms of like just MLOps and scaling as well on, on multiple machines.
[00:40:58] Alex Volkov: I think, Omar is there anything else important that we missed before we move on?
[00:41:02] Omer Sanseviero: And that was pretty much it. I just wanted to say that there's also a chat model that they released with this that was pretty much it.
[00:41:09] Alex Volkov: Awesome. Omar, thank you so much for joining folks who don't know Omar's Chief Lama Officer in HuggingFace. I will mention this every time the Omar comes and thank you incredibly for your experience folks should definitely follow you because I added to the show notes, the deep dive from the deep seek paper that you shared.
[00:41:24] Alex Volkov: And I really appreciate your expertise here and the breakdown. Thank you Omar, Nistan, go ahead.
[00:41:30] Nisten Tahiraj: Yeah, just for the GPU poor out there just wanted to say that when you do PyTorch it does not support FloatingPoint 16 layer norms. [00:41:40] So if you're using MergeKit with PyTorch and want to do it all on the CPU, You got to convert the weights you're going to merge to floating point 32, you can do it without a GPU, just for people out there.
[00:41:54] Nisten Tahiraj: So if you're going to merge get very large models, you're going to need to have all the GPU memory and that could be more than 130 gigs in some cases. Yeah, just one thing in case anybody's struggling to use MergeKit.
[00:42:07] Alex Volkov: All right. Thanks, Nistan. We're still in the open source, like we've been an hour, but there's a lot to cover in open source. The last thing I wanted to briefly, briefly mention, really, we don't have time to go deep into this, that Argiya and John, I think you mentioned Argiya as well.
[00:42:20] Alex Volkov: Argya they have a tool called Distilled Label and they do like a bunch of like cleaning of DPO datasets and they actually took the, if you guys remember, we talked about Intel released a fine tune of a fine tune of news based Hermes model and they released a neural Hermes. Based on, I think they trained it on like some custom gaudy hardware or something like this.
[00:42:41] Alex Volkov: And then Arge, who's the company that now does DPO, they released a fine tune of a fine tune of a fine tune and they used this still label tool to build a DPO dataset. And then they did some stuff with ratings and critiques and it took them around three hours. , the original data set assumed that GPT 3 or 4 responses were always best, but Argilla did like a comparison with their tools and found that's not always the case.
[00:43:05] Alex Volkov: And they had some numbers that I put in show notes, but they did their data set confirmed like 4, 000 pairs had the same rating and 7, 000 pairs were unchanged. But there's like around 2000 times they rejected the response that was preferred from GPT 4, which basically like a, another cleaning of a data set.
[00:43:21] Alex Volkov: And they just showed that like with 54 percent fewer DPO pairs, they could beat the already like great performance of that model. So super cool. And check out our GIA. They're doing a bunch of stuff in open source. I know that we in Weights Biases use their GIA to also like. Create and look at data sets definitely worth a shout out.
[00:43:38] Alex Volkov: And I think in open source, that's all. I think we're finally moving to the area of big companies and APIs for which I don't have a transition music.
OpenAI big week - GPT store, Team tiers and personalization announced
[00:43:47] Alex Volkov: But I think the next big topic to cover is the OpenAI had a big week as well, and I'm just going to briefly cover this and then maybe some folks here on stage who already have a GPT.
[00:43:57] Alex Volkov: OpenAI finally, we mentioned this last week, OpenAI finally released the GPT store. If you guys remember back in Dev Day, they announced GPTs as a concept, but they also said that, hey not only GPTs are a replacement for the previous plugin era, GPTs are also a way for me as a creator, who's maybe non technical, to create a GPT and share it with the world.
[00:44:17] Alex Volkov: And plugins didn't have a shareability but they also announced that they're going to have a marketplace for GPTs. Not only that. They will also do some revenue share. So they finally announced and released the GPT store. It's now live on your chat GPT if you pay for premium and that store now has trending GPTs and also shows how many people did, how many chats with your GPTs.
[00:44:39] Alex Volkov: I've mentioned previously on Thursday, I, that I had one idea that's called visual weather GPT, I'll edit the show notes as well, and that's. That I'm happy to say the past like 5, 000 chats now, and it was for a brief moment, it was number six in the lifestyle section, but it disappeared. And so it's pretty cool as a launch.
[00:44:57] Alex Volkov: Many people, as always on Twitter, many people have different takes about whether or not this will succeed for OpenAI, but I just want to shout out the incredible work they did. It looks smooth. They did have some, looks like downtime on interference because it looks like many people started using. Just because there's a bunch of GPTs I personally am using some GPTs like constantly, and I wanted to also, as well, welcome to the stage.
[00:45:21] Alex Volkov: We're going to talk for, and for a deeper dive, but you also had the GPT that's been very, so I think Kru had the GPT, right? Could you talk about like how that went and what made you create it?
[00:45:32] Joao Moura: I gotta tell you, when GPTs came out at first, I was like very curious to get, to give it a try. And one of the main use cases that we had was basically that And in Clearbit, like we had a lot of issues when going through support, trying to have to figure it out, like, how do I fix this issue?
[00:45:51] Joao Moura: Other people have fixed it in the past. So we internally had created like a GPT that knew like how to go about this issue. So if you had any recurring problem, this GPT could help you out. And then in the midst of that, I was also writing some docs for Clear AI. Hey, it would be pretty cool if you didn't have to explain to GPT every time what this library is and how it works and all that.
[00:46:16] Joao Moura: I put together this and fit it with all the documentation that I had written about Career. AI. And then I started using it myself and it was so good. Honestly, a lot of people that don't like, like going and reading through the docs. You can just like chat with it, and it knows everything about it.
[00:46:34] Joao Moura: You can center the conversation around your specific use case, and that just makes like things so much easier. I think every library out there should have something like this.
[00:46:45] Alex Volkov: Absolutely. And I will just mention I will be remiss if I don't mention that we also have something like this. It's called WandBot and we have trained a rag model bot and used to previously work in, in, in our Discord and internally in our Slack. And Morgan, who's in the audience. Recently added this to GPT's and at least for me, this is like the preferred way to use this because I'm like, I don't maybe don't want to switch to slack and it's built in and we use the kind of the backend APIs and I've used the crew AI one as well.
[00:47:12] Alex Volkov: It's definitely more shareable and I hope that open AI kind of keeps doing this marketplace. I will let you know guys, if I made any money based on my visual weather GPT and I'll definitely try to keep you in the loop in terms of what's the revenue share like? I will say this, I will say this, and I don't know if you guys notice there's a bunch of copycats already.
[00:47:30] Alex Volkov: There's like many people complained already that they have like the top GPTs that now, because the search is one of the ways to discover GPTs. And obviously as every marketplace happens, every marketplace has this, like a bunch of issues that people try to like hack into. And so this already has a bunch of copycats.
[00:47:48] Alex Volkov: I think one of them is GPT something. That's just like literally take the most important GPTs and copycat them and do pretty much the same. GPT is also worth mentioning. They don't hold any information that you have in there as a secret. If you have enabled Code Interpreter, Advanced Data Analytics in your settings for your GPT.
[00:48:07] Alex Volkov: All the files that you upload, even for the REG stuff, can be downloaded. And I think, Umesh, we mentioned this you mentioned this in your spaces, that this is this is something that people should definitely know. And also so discoverability is great, but definitely the ease of copycatting is interesting and whether or not OpenAI will how should I say, treat this properly?
[00:48:26] Alex Volkov: The only thing that I think that they did very well is that you have to verify your domain in order to GPTs to be like featured on the store and verification of domain is not super simple for bots to do. So there's one or two domains that are trying to copycat everything. Hopefully very easy for OpenAI to clean this out.
[00:48:43] Alex Volkov: An additional thing, the OpenAI released. This week, and okay, so they released something that we haven't played with, but they released personalization, which I'm waiting for, and I'm sure that 24 is going to be the year of personalization but they haven't let me play with it yet. They just announced, and I just saw the announcement, but basically They released a thing that will keep your GPTs remembering facts about you and about the stuff that you shared throughout sessions, right?
Personalization is coming to GPT
[00:49:07] Alex Volkov: So as Xiao just said it's annoying sometimes when you just come to chat GPT and you have to re say again, Hey, my name's Alex. Hey, I work at Weights Biases. Hey, I have a kid like this year old, blah, blah, blah. So personalization is coming to GPTs. I think it's going to be like a significant moat on their part because they will start keeping the memory for many people.
[00:49:25] Alex Volkov: This may. Not be happy, but I, or may not be the best thing, but I'm pretty sure that it's very important for our AI system to be very helpful for us to, for them to actually remember. And for me not to have say the same things again. So definitely you can add some to the custom context, but it's not enough.
[00:49:42] Alex Volkov: And it's going to be very interesting how the personalization stuff happens. So this was released by them to, I think a few people, it's not wide. The thing that was wide is the JGPT team. Worth mentioning that Chadjib team kind of tiers were released and it's like [00:50:00] around 25 a month per user for minimum of two users.
[00:50:03] Alex Volkov: If you pay for the annual plan A confusing thing there was that like we now get access to G PT four with 30 2K context window and I'm sure that I've had access to 30 2K before because I know for a fact that I used to paste all the transcripts for Thursday I into check G pt. I would say, oh, too much context and this is way more than the eight K that g PT four.
[00:50:24] Alex Volkov: But then after they released the turbo, I know for a fact that I was able to paste all of the transcripts. So I don't know if they what's called the retconned. I don't know if they went back and removed some of the previous features that we had in the pro tier to make the GPT team tier better.
[00:50:38] Alex Volkov: I don't know. And there was a discussion about this, but an additional thing that worth mentioning that they offer in the GPT team is that the ability to. Create and share custom GPTs within your workspace. So that's super cool, right? Because we know that all the GPTs are public. And if you do want to have a GPT with like custom information, but you don't want people to copy it, the ability to share it within your teams, I think is super cool.
[00:51:01] Alex Volkov: And I think this is the major play here. And an additional highlight is they're not doing any training or your business data or conversations in the GPT team. Which does mean that if you pay for pro, they do train on your business data and conversations if you just use this via the UI, which triggered some people and some people came into my comments and said Hey, there is a way to tell OpenAI to their support thing you can email them and there's like a self serve tool that you can actually ask to opt out of training.
[00:51:30] Alex Volkov: So even if you don't pay for the GPT. team tier, you're able to go to OpenAI and say, Hey, for my account, please do not train on my inputs or data. And it's possible to do so definitely, if you're concerned about this, reach out to OpenAI, to the support but the GPT team kind of highlights this as a business use case, you pay for that and you get this.
[00:51:53] Alex Volkov: They have admin console, workspace, team management, and early access to new features, which sounds to me just marketing until I actually see people on the team tier, get something that I didn't get on the pro tier. But hopefully we'll get a team tier as well. And then I'd be able to get early access to new features and improvements.
[00:52:09] Alex Volkov: I think that's most of the things in from OpenAI. And I think the confusion about like training on your data was the main point of confusion. Umesh any thoughts that I missed on the GPT OpenAI launches before I move on?
[00:52:21] Nisten Tahiraj: Just they are saying higher message caps, but not mentioning any numbers. So there is a lot of ambiguity.
[00:52:27] Alex Volkov: Oh, yes. I think they mentioned somewhere, I think Logan did and hopefully we'll get Logan next week and we're gonna check about this. I think 2x the message cap. And I think they lowered our message caps in order to get the 2x on the team, I'm not sure. I saw somebody mentioned that we had 40
[00:52:43] Alex Volkov: Hours or something, and now we have 30.
[00:52:45] Alex Volkov: I will go double check, but definitely higher message caps. And also, the caps are not together, right? So like per team member, you have your own caps. It's not like everybody gets the same amount of chats. And so those are definitely split. Thank you for that. All right, moving on real quick.
This weeks buzz - news from Weights & Biases
[00:53:00] Alex Volkov: I want to introduce a new segment here. I don't have music for it, so I'll do the Christmas music for it for now. Christmas music! I want to introduce a new segment called This Week's Buzz. This Week's Buzz is like a little play of words on the 1DB Weights and Biases. This Week's Buzz has been a segment in the newsletter where I talk about what I learned in Weights and Biases every week.
[00:53:21] Alex Volkov: Here, I think it's very well worth mentioning that this week I'm flying to San Francisco to AGI house. I think I mentioned this before, there's going to be a hackathon there called fine tuning versus rag on open source models, where this question of whether or not what's the best outcome if you do rag and then do a true logman generation for your applications or you fine tune on some data or maybe both.
[00:53:45] Alex Volkov: So there's a bunch of folks there that are going to join AGI house and try to figure this out. And Weights Biases is a co sponsor of this hackathon. Together, Compute is a co sponsor of this. I think they're the main organizer and also LengChain and Harrison's going to be there. And if you guys know, we talked about together before, Mamba is from TreeDao who's working together and the Hyena Architecture we've talked about also comes from Together.
[00:54:08] Alex Volkov: They're incredible folks. And recently some friends of the pod also joined there, so I'm going to be very excited to join there and go there. And I think I have a talk that I need to finish the slides for, but I want to invite everybody here who is in the area and is a super cool hacker and wants to join to please join.
[00:54:25] Alex Volkov: And I'd love to see you there. And we have some cool prizes on there as well. And I'm probably going to do some live streaming as well from there. So definitely feel free to follow me if you haven't yet. For that. Probably on the main Weights and Biases accounts. And I think that's the whole announcement.
[00:54:39] Alex Volkov: I'm very excited to go again and besides the fact that my flight just got canceled as I was like going on the space and I need to figure out a new flight, but I'm going to be there on this Saturday with Together and LengChain to do this hackathon. It's going to be super, super cool. And hope to see some ThurdsAI folks from there in there.
Luma AI - Genie is available on web and mobile
[00:54:56] Alex Volkov: I think, folks, I think that's mostly No, last thing that we need to cover. We have Arthur here on stage. Before we get to the interview with Zhurao and CrewAI I think we need to finish off. LumaAI, who we have representatives of on stage, but we knew Arthur before he joined, has released their Genie which we've talked about before.
[00:55:14] Alex Volkov: Genie is their kind of bot that generates text to 3D. And we've played with this on discord before, but now it's possible to play around on the actual web and in mobile. And Arthur, I want to welcome you. Thank you so much for patiently sitting here. First of all, congrats on the release. And could you tell us more about the genie online?
[00:55:32] Alex Volkov: And I think you have some more news for us.
[00:55:34] Arthur Islamov: Yeah, thanks. First of all, I would like to say that Luma recently has raised Serious B and we're expanding the team.
[00:55:40] Arthur Islamov: So if you want, yeah, if you want to join us to work on multi modal foundation models. You are welcome to work with some amazing people. And yeah, about the Genie, we have did quite a lot of improvements since our first initial release on Discord.
[00:55:58] Arthur Islamov: And now you can generate it on the web and in our iOS app. And we are soon releasing API Access. So if you want, you can message me and to get some preview of that, but I guess soon it will be generally
[00:56:15] Arthur Islamov: available.
[00:56:16] Arthur Islamov: And yeah, I guess I can just tell that we did quite a lot of improvements with topology and some other feedback from the community since our first release, and people find it very cool to generate some assets for their games or something.
[00:56:31] Alex Volkov: Especially via API, I assume that's gonna be great, right? I know many folks UIs are nice, but people are programming different prompts and techniques and different things, and then they see assets and everything. So it's super, super cool. I played with the genie stuff in Discord, definitely, it was like, cool, hey, create a frog, and then it gives you a 3D frog.
[00:56:47] Alex Volkov: But now they actually look super cool. So one of the teammates on our weights and biases is Polish. And I generated like a Polish bear for them. It looks really cool. Higher quality as well. There was an option to upgrade your models as well.
[00:57:01] Arthur Islamov: But yeah, I am working on the foundation models too. And another thing to mention, that it is completely free now, so you can play with that as you like.
[00:57:12] Alex Volkov: Play and then download the actual models in a bunch of formats. And then, I think we also mentioned Junaid in the audience mentioned that you can 3D print based on the obj files or something like this. So you can generate and 3D print from what a world we're living in, right?
[00:57:25] Alex Volkov: You can type something and get a 3D image. Super cool.
ByteDance released MagicVideo paper - text to Video with higher quality than Pika/Runway
[00:57:28] Alex Volkov: All right. Thank you, Arthur. And I think in this kind of area, we also should mention that ByteDance also released like video generation called magic video. They only released the paper and the project, no code yet. So you can't like actually play with this.
[00:57:41] Alex Volkov: But if you remember, we've talked about stable diffusion video and PikaLabs and motion. Oh, sorry. Forget the runway ML also generate video. So now it looks like ByteDance, the company behind TikTok who has. Previously been in the news saying that they bought like a lot of GPUs are releasing very beautiful videos.
[00:58:01] Alex Volkov: They really breathtaking in how beautiful they are. So definitely worth checking out. I'll put this in the newsletter. I think we ran through all the news and I think we're like a little bit behind, but I think it was worth it. And I'll do a little like transition segment. And then we're going to talk with throughout.
[00:58:17] Alex Volkov: And thank you for sticking with us. The [00:58:20] news segment is done, but the conversation is not done. The Thursday is not over yet. And we have an exciting guest today. So I'm going to introduce João in a second.
[00:58:28]
[00:58:28] Alex Volkov: Joao Moura joined us. He's the author of Crew AI, an agentic framework he built that uses both GT four and other APIs, but also can work with local models and is able to spin up multiple agents that work together in the crew, get it crew ai to execute on tasks. He built it for himself and we had a beautiful conversation of how in the field of a bunch of agent frameworks that are popping up, he was able to create one of his own and it was.
[00:59:01] Alex Volkov: Simultaneously, the most trending GitHub project, and number two on Product Hunt as well. Surprising to him, he didn't plan on this, and it was a great conversation, definitely worth your time, and it's going to be released separately together with the John Durbin in one episode coming soon. Back to news.
[00:59:19] Alex Volkov: Alright folks, I think the last thing that we, I promise we'll cover, and we didn't get to, and now we have a friend, a guest, that we're going to cover together, is the Rabbit hardware. So we don't often talk about hardware, we do sometimes.
[00:59:33] Alex Volkov: And we've talked about the HumanePin, obviously. Pre order one. I'm very excited about it to get my hearing pin. And this week was this, the week in CES. There's a bunch of, everybody talks about AI over there. Obviously this is like the hot new thing. So hardware now is like open skies and people try to build.
[00:59:49] Alex Volkov: And the kind of the thing that broke the internet was the presentation from The Rabbit Company about their R1 product and also their large action model, which they went into deep about like the differentiation between large language model and actions. And so I want to introduce Dan Jeffries, Dan, right?
[01:00:09] Alex Volkov: I almost said Daniel, but Dan Jeffries to the stage. Welcome Dan.
[01:00:13] Dan Jeffries: Thanks for having me. Can you hear me
[01:00:14] Nisten Tahiraj: okay?
[01:00:15] Alex Volkov: you're coming through great. Then we met I think a year and a half ago at the Stability AI event when you guys launched in San Francisco. You're currently, you said, Managing Director of AI Infra Alliance and CEO of Kentorus, which is a new stealth. Anything else I'm forgetting?
[01:00:32] Dan Jeffries: No, those are, a lot of people known me for my blogs and all that kind of stuff over the years, so that's but that's about it. Yeah, those are the big ones.
[01:00:38] Alex Volkov: I personally know you from your like impeccable dressing attire. And when you introduced me, it's Hey, I'm Dan Jeffries, as though I was supposed to know who you are. But then I found out who you are and like, started following you and I really appreciate your takes. So I wanted to cover with you the Rabbit Launch because I pre order one.
[01:00:53] Alex Volkov: I know you as well, but you had a nice breakdown kind of thread and I wanted to hear your thoughts. What do you think about this Rabbit hardware device?
[01:01:03] Dan Jeffries: Look, I think,
[01:01:04] Dan Jeffries: I, it was one of the first presentations that I've watched completely since maybe the iPhone one. It's a 30 minute presentation
[01:01:10] Dan Jeffries: and that, I don't know. Who the hell has time to watch that anymore, right? But I watched it, and I was going through a lot of it, and I was, there were a couple points in time where I was like, Bullshit!
[01:01:20] Dan Jeffries: And it was both an expression of that's really awesome, and I'm a little skeptical, right? And so like I did end up buying one because it's a low price point, right? It's not really like buying Apple's giant spatial computing boondoggle, right? Where you're like, let me buy this thing, it's got two apps, and then all of a sudden you're like, shit, this thing's sitting like in the corner, I'm never going to use it again.
[01:01:40] Dan Jeffries: This is 200 bucks and it's basically a glorified Kickstarter. I'm not going to worry about
[01:01:43] Nisten Tahiraj: it, right? But,
[01:01:45] Alex Volkov: they also mentioned that there's no subscription.
[01:01:49] Dan Jeffries: yeah, and let's talk about a few of these things, right? Number one is a lot of people were, like, talking about the idea of running locally, and I think You know, Kriya has talked about those things. And I'd love a bunch of stuff to run locally. And you see a lot of the people running Mixed Real and all these things locally, but it's really hard to run these things locally.
[01:02:04] Dan Jeffries: And I can tell you that for a 200, device, there's 0. 0 percent chance that it's running locally. A lot of people seem to think that it was, and I just don't buy that. And there were a lot of things as I went back and looked at it, I'm like, I don't, I'm rooting for these folks because I really want this thing to work.
[01:02:20] Dan Jeffries: But I'm not really buying it. And maybe the first one that kind of came to mind is the lamb itself. And there's a couple of things that don't pass the sniff test for me. Number one is the term,
[01:02:30] Nisten Tahiraj: neurosymbolic. Okay, so that is like
[01:02:32] Dan Jeffries: a favorite of what's his name Gary, Gary Marcus, he's the big there are a lot of people who think NeuroSymbolism is a great idea, but it's never actually worked in production. So I'm a little skeptical from that standpoint. The other thing is, there have been people who have been working on it, so I think Prue, the guy I followed, said earlier that they were, that people are working on that.
[01:02:50] Dan Jeffries: There is actually an open source one that's that my favorite paper of last year was AgentTuning. And they open sourced it, they trained it on a whole bunch of web tasks and clicking around things. And they took Lama 2 from being, like, 2 6 percent accurate, depending on the version, to something like 80 plus percent accurate on some of the, on some of these tasks.
[01:03:08] Dan Jeffries: There are people working along this line, and so I, I do think it's the correct line, but the fact that it was basically 100 percent accurate, it was doing it at frickin lightning speed, there was no latency on anything, both from the conversation to how fast it was making decisions makes me really freaking skeptical of like how much of this thing can actually eat.
[01:03:26] Dan Jeffries: I don't know how much of it is actually working because it feels like they solved multiple machine learning problems in one. And maybe the one I'm most skeptical of is when they showed it mid journey at the end after a minute. Now, I want to, I want everyone to remember that a week ago there was the guy from the new robot company or whatever who was like, we trained the
[01:03:43] Nisten Tahiraj: model.
[01:03:44] Nisten Tahiraj: Yeah. Yeah. And he was like,
[01:03:45] Dan Jeffries: wait, we trained it on, human examples. And it was like, By the way, 10 hours of training on human examples so that they could put the fricking Nest cafe thing in there and click the button. Okay. So like
[01:03:57] Dan Jeffries: it
[01:03:57] Alex Volkov: context for folks who have missed this, who don't live on X as much as we do I don't remember the company, but Brad Atcock from the robotics company, they announced something on X in the post that says, Hey, this is the ChatGPT moments of robotics coming tomorrow, like the breakthrough, and then they show the video of their bot basically making a Keurig cup of coffee shoving this like little Keurig cup.
[01:04:18] Alex Volkov: So not like a, even an espresso machine, I think it's like a very basic kind of coffee machine. And then a bunch of folks showed that they previously done this not to take away from their robot, like hopefully, it does learn, but yeah, definitely it didn't feel like the Chajapati moment for robotics based on that example, at least from what I saw.
[01:04:36] Dan Jeffries: That's right. And like it's, I just looked it up. It's figure robotics. And by the way they're real. Yeah. They're real AI researchers and they have a real robot. And this technique of training it based on example is a real technique. And there's, if you look at the stuff outta deep mind, right?
[01:04:49] Dan Jeffries: And the progress and compress and all these kinds of things they're working on or when they were working with those soccer robots and they were just basically training them on the basic idea and then letting them get around and, kick stuff and figure out their own strategies.
[01:05:00] Dan Jeffries: There's a lot of this stuff that, that is really advanced, right?
[01:05:03] Alex Volkov: Yeah, but bringing you back to this happened like a week before, before Rabbit.
[01:05:09] Dan Jeffries: So
[01:05:09] Dan Jeffries: that's like basically 10
[01:05:10] Dan Jeffries: hours for it to basically put in the cafe, thing in the top of the machine and press the button to pour the coffee, right? Not even pick the coffee up and hand it to the person.
[01:05:18] Dan Jeffries: And then they basically showed it mid journey at the end of the day, in one minute, and then we're like, okay, go generate a bunch of stuff in mid journey and it did it perfectly. And I'm like, okay, you've just solved multiple machine learning problems in one, one go. Like one is you just gave it a single shot learner.
[01:05:35] Dan Jeffries: With one example and it basically learned it perfectly and then, it's like, how are you updating the model behind the scene? Are you like making a lure for everyone? Are you fine tuning it? If you fine tune something over time, it's going to degrade, like how many things can you add to it
[01:05:49] Dan Jeffries: et cetera.
[01:05:50] Dan Jeffries: So it made me
[01:05:51] Alex Volkov: Yeah, Daniel just one second, for folks who haven't seen the video the setup I think was something like this, they had what I assume was a Chrome extension running on the browser, because I don't know how else this thing records, it was a multi modal one, so it was recording the screen, but he was also talking, and I wasn't sure if the guy was talking for us for the video or actually recording.
[01:06:11] Alex Volkov: And it looked like he was recording audio of what he actually does into the recorder. And what he actually did was like, Hey, I'm going to this mid journey server. And he was like very explicit about that. And so he had the software that sees what he does, probably Chrome extension, because I think he used the web version of discord which by the way, mid journey specifically.
[01:06:32] Alex Volkov: It's against the terms of service of Midjourney, so we'll see if they kick them out. And then he basically went through generating an image on Midjourney, and then [01:06:40] he trained this thing of theirs, this action, and then he was able to use the Rabbit kind of hardware device which is connected somehow to this learning, and to perform the same action.
[01:06:50] Alex Volkov: And I agree with you, it's It seemed that they have like several things that they solved, and additional things that they solved. First of all, that was sped up, right? The performance, because they were from Mid Journey, etc. The cool thing that I saw was they were able to pull out the images as well, so that was super cool.
[01:07:04] Alex Volkov: They were literally able to lift the images from the output of Mid Journey, put them in the Rabbit interface. That looked cool, though I don't know if it generalizes. What else did didn't connect with you, even though you did buy one?
[01:07:17] Dan Jeffries: Look look,
[01:07:17] Dan Jeffries: the thing is. If you work in machine learning, or you've been around machine learning a long time, or you work in the agent space, or whatever you know how hard these frickin problems are. Cause I've been looking at all these problems probably pretty hardcore for the last 8 months on an individual basis.
[01:07:31] Dan Jeffries: Planning a trip, I've tried like 10 applications, actually, that I've tried to plan a trip and they all suck, right? And it's hard is it gonna go read TripAdvisor and just pick the top stuff? It, it picked the trip in three seconds. Like, where? Did it read anything? Like, how did it do that?
[01:07:44] Dan Jeffries: So there's a lot of stuff that just doesn't add up. I think the thing is, I went against my better judgment that I just want this thing to be real because I think everybody wants the functionality in this thing to work. In other words, if I could say, you know what, I've got to plan a trip with my wife coming up.
[01:08:01] Dan Jeffries: She's a master planner. She's a project manager. She lives and breathes by this stuff. I'm like, Oh my God, I got to plan. Six days worth of like lunches and all, and I'm like, like driving myself crazy with it. So I would love to have, a HER like agent that just goes and does part of it for me.
[01:08:15] Dan Jeffries: Even if it's 50 percent of it and I have to fix it, right? I'd love for it to be able to go learn an application and do it pretty much flawlessly. Because again like Francois Chollet said, look, AI will become our interface to the world. And this is a chance
[01:08:27] Joao Moura: for us to take back,
[01:08:29] Dan Jeffries: Our, the way that these machines work over time.
[01:08:31] Dan Jeffries: If you didn't think about Twitter. They take away your engagement over time, or they take away your ability to connect with people, or they show you what they want you to see. Airbnb shows you what it wants you to see. And I've learned a bunch of tricks, for instance, over time on Airbnb, where it's okay, I'll go into the reviews and I'll search for noisy and loud and dirty and filthy, right?
[01:08:48] Dan Jeffries: And it's I see too many of those gone. If I see a TV up on the wall, not in line, with the couch, then I know it's a tiny piece of shit, because nobody puts their TV like that. Like you always put it in front of the couch, not up on the wall near the kitchen, right? So I'm like, if I could have an agent that I could say here's my expert knowledge of having traveled a lot go filter it in this way and give me like the top five places or whatever, and make sure they're, Complete Pure.
[01:09:12] Dan Jeffries: That's amazing. Everybody wants this thing. Everybody wants it to be real. And even if they've solved 50 percent, I'd be happy with this thing. I'm going to be surprised, quite honestly, if they solve 10 percent of this, and it's not just like a It's not just, like a glorified Kickstarter.
[01:09:27] Dan Jeffries: That said, I'm freaking rooting for them. I
[01:09:30] Nisten Tahiraj: really am. I want this to be freaking true because I want this goddamn device. I'm trying to build it in software. Crew AI is trying to build it in software. Other people are trying to build it in software. Thousands of other people are trying to build this damn software.
[01:09:42] Nisten Tahiraj: So is OpenAI.
[01:09:43] Alex Volkov: I wanna hold a physical device that was designed by Teenage Engineering that looks like a Tamagotchi that has AI built in, multi modal AI, as a video camera, I can point it at things and ask it to do real world tasks, and I definitely wanna connect it to, whatever, compare it to the HumanePin, and For sure.
[01:09:59] Alex Volkov: I think Umesh, you had some more concepts as well. Then thank you so much for hopping on first time on Thursday as well. And just we're literally like at a two hours mark. And I also want to recap real quick Umesh, you had also some thoughts and you were telling me about Rabbit before. I want to hear from you about Lamb specifically, and I want to finish with some thoughts of my own of why I got this.
[01:10:18] Alex Volkov: I also connect with Dan, like I want this device to exist, so might as well vote with my wallet, right? Go ahead Umesh.
[01:10:25] Umesh Rajiani: Yeah, very quickly. Basically it plugs into what Dan was saying. That basically the biggest challenge that you have when specifically leveraging LLMs and we are trying to do that is, is the amount of data that you need to pass in for automation, any kind of automation, because LLM basically you have to explain the actions into language and that just goes on into a number of tokens and what they seem to have mastered, we haven't seen the actual proof yet, but what.
[01:10:51] Umesh Rajiani: They seem to have mastered is leveraging LLM only to identify the actions that need to be taken and be the, because the actions are already encoded into lamb. So the large action model you don't need to, you don't suffer from the latency or the generation that you have to wait for, from LLM. So that seems to be the key that they seem to have.
[01:11:14] Umesh Rajiani: And the reason I'm. Bullish more is because not only because of the way they have demonstrated the device but all and the architecture that they have shown which on theory on paper looks really strong but also in terms of team because we know Khosla or Khosla Ventures have actually invested in quite a few, device startups like DoorDash and all And they've been quite successful.
[01:11:36] Umesh Rajiani: And Jesse is not only like they leveraged team engineering, but he's on the board of team he does have,
[01:11:43] Alex Volkov: CEO,
[01:11:44] Nisten Tahiraj: yeah, the CEO, he's on the board of team engineering. So it's not they are coming from nowhere. There is a significant high profile team behind it.
[01:11:52] Nisten Tahiraj: So that doesn't mean it doesn't ensure, but overall like ingredients are there to be successful. So let's see.
[01:11:59] Alex Volkov: rooting for them. They sold out the first, I want to say, they said 10, 000 devices. They didn't expect it and they reopened like another batch. So they did make, what 2 million, 6 million or something. I'm not good at math, but They made a bunch of like money on the first day, which was incredible. I think they managed to trigger the system the ecosphere on Twitter.
[01:12:20] Alex Volkov: Many people said, Hey, why wouldn't this just be an app? And many other people said, Hey, I just want to own a device by teenage engineering for 200 bucks. No subscription seems very interesting, the speed with which it performs this task seems triggering for people who remember the Gemini release and then end up being like an edited version and Gemini doesn't really understand video.
[01:12:41] Alex Volkov: I heard like all these takes. I bought one three minutes into the video. First of all, 200 bucks, like I've spent more than that on one evening in a restaurant. Second of all, if I end up giving this device to my kids, this is the perfect If you think about this from this way, this is the perfect device for kids, for parents who have kids who they don't want to buy iPhones for, because then Instagram will rot their brain.
[01:13:04] Alex Volkov: This is the perfect device for that. This is just like a, it's like a Tamagotchi. You can talk to it, the rabbit is super cute. There's one simple interface, it's voice. Kids can hold the button and talk. I think for older folks, this could be like an interesting thing as well. Will Rabbit be the one, will it be the next iPhone?
[01:13:20] Alex Volkov: I don't know. But I do know for the folks who said, hey, why wouldn't this just be an app? It's because Apple It has a monopoly and some people are using AI to break out from this monopoly and AI can replace the interface for many of these tasks that you want to do. And I'm really happy that folks are trying this.
[01:13:36] Alex Volkov: I'm rooting for Avi Schiffman from TAB. I plan to visit Avi again in San Francisco. Hopefully he's going to be there. I'm rooting for Humane, which I pre ordered and I'm very excited about and I've interacted, hopefully Humane folks will come on ThursdAI at some point. I'm rooting for Rabbit. I don't plan to buy more of these devices this year, but Umesh, as you mentioned, I may not be able to resist.
[01:13:55] Alex Volkov: I'm rooting for all of them if they open source some stuff. So we saw some effort and a lot of excitement from Killian Lucas from Open Interpreter to say, Hey, let's open source a device like this. Let's buy a Raspberry Pi. I'm rooting for the open source area as well in these devices, because I want to live in the future where AI is embodied and lives with me in the physical world, sees what I see and is able to just answer me.
[01:14:17] Alex Volkov: Then like you planning a vacation, I hate this. I'd love to talk with a hundred people live on X rather than planning a vacation. I know for some people that's scary, but for me, this is what I like to do. So I would love a device that helps me do this. One last thing for me from Revit is something that I understood is that, It's a remote control for stuff on my Mac, if indeed it's able to do stuff like it did with MiJourney.
[01:14:40] Alex Volkov: It's essentially obviously it doesn't use any of my MiJourney credentials on their servers, right? So it actually probably ran the MiJourney on my specific device. So if I have a physical. Remote controlled AI that can run stuff on my Mac. I'll buy another Mac just for that. [01:15:00] And I'll learn and teach it a bunch of stuff.
[01:15:02] Alex Volkov: And I'll just be walking around the house and say, Hey, schedule a Thursday iMeetup or whatever. Release an update or something like this. I'm very very excited about some stuff like this, so I'm looking forward for my Rabbit, so hopefully it will launch soon. This wasn't a paid promotion, I know they probably paid some folks.
[01:15:18] Alex Volkov: I didn't get like a cent from Rabbit, I just really like the AI devices.
[01:15:22] Alex Volkov: ,
ThurdsAI ending recap
[01:15:22] Alex Volkov: and then we're going to meet you here in next week. With that, thank you so much for joining.
[01:15:27] Alex Volkov: Check out the show notes. They're coming after this. Cheers, everyone. Have a nice weekend. Bye bye.
[01:15:38] Nisten Tahiraj: Bye Alex. Good to know.
Share this post